Podcast
The AI Big Bang: Exploring Government’s Growing Universe of AI Applications
As the public and private sectors identify which innovative AI solutions to adopt, they’re faced with increased consumer expectations, ethical considerations, and questions about operational needs.
The AI Big Bang: Exploring Government’s Growing Universe of AI Applications
Transcript
Moderator, Question 1:
James, come to you now as the founder of Striveworks, to ask the question about the universe of AI as it’s being discussed in the public forums. There were even calls from a thousand technology leaders and researchers, including Elon Musk, that said artificial intelligence needs to be paused, naming one of the primary concerns, accuracy and accountability, and of course nefarious uses. So, when you heard that, what did you think? As someone who works in AI, what were your reactions to that?
Jim Rebesco:
Yeah, it’s a great question, and I’m not a policy expert. So, with that caveat upfront, I’ll kind of say as it directly related to that, candidly, I struggled to see how that particular petition really laid out a constructive alternative, and I think that’s always an important kind of element within a policy discussion. And so, as I kind of expand that question to think about what is responsible AI and why does it matter, I think the first thing that we all need to keep in mind is that the notion of responsibility and accuracy and accountability kind of contains within it a very natural human tradeoff. And the things that a 737 pilot would do when flying people from Austin to Washington, DC, is very different from what we’d consider responsible for an F35 pilot because they’ve got a profoundly different platform and they’ve got a profoundly different mission to accomplish with it.
So, with that kind of anchor in the back of our minds, I’ll go back to something Pat said. I thought he framed out a bit of the “why” around AI very, very well. And they said that what Babel’s doing is focusing on NLP, but they’re trying to provide structure to unstructured data. And I think from our lens very broadly, that’s not just kind of what AI and ML do, but mathematical functions in general. And as we talk about data ETL processes or even RPA or robotic process automation, these are all kinds of means and methods. And from my perspective, they are ways of transforming data from a less useful format into a more structured and useful format that people can query, ask questions that are relevant to them and their business, their organization. So, if we are willing to live under the big tent of all these things we’re talking about are ways of transforming data in some way or shape or fashion for later query search and interrogation, then from my perspective, doing this responsibly really has to be a whole system approach.
And for us, that’s people, that’s process, and that’s platform. Those are the three pillars. I think we’ve heard from some folks already today who’ve spoken, I think very thoughtfully and articulately, on the people piece and how this is not just an element of training, this is also an element of assessment, understanding where people’s data literacy or skills rest and making sure that they’re able to interact with, develop, or interrogate AI systems in a way that’s commensurate with both their need and their kind of training and background. A process, I think, is another key point there. And this is the development of analytics, the development of AI, if there’s an element of it that’s an art, it’s an industrial art, and we need to—and this kind of comes back from my experience—it’s very important not just to be able to do this in a systematic and defined way that lets you do two things.
One, it lets you scale. That’s very useful, being able to do this at broad enterprise scale. And two, it allows you to talk to that regulator, that kind of third-party auditor in a way that says, hey, between data and decision, I wasn’t just kind of doing jazz between data and decision. I followed a thoughtful and quantitative process, and I can kind of tell you what that was in a way that allowed you to feel comfortable on where this model came from and what it was used for. The last part of that, I’d say, would be platform. We heard a little bit about technology platforms. Why do platforms exist for reasons? They exist to accelerate your time to market on products. The second reason is that they exist to reduce the total cost to own and operate those analytics once they’re developed. The third reason is to lower that skill barrier to get people productive. And the fourth reason is to enhance security. And I’d submit to you that if you have an organization that can kind of touch those three pillars, right? Being thoughtful about their people, being thoughtful about their process, and has that platform to do that, you’ve got actually a very nice framework to allow AI/ML, RPA processes to not just be developed, but to be deployed at scale within a responsible framework.
Moderator:
That’s an interesting way of putting it because you’re absolutely right. I mean, I don’t think everyone is going to agree about how AI ends up manifesting because there are so many different versions of what it is and how it’s used. So, thank you very much for that.
Moderator, Question 2:
James, from Striveworks, analytics is a growing area of AI using all the data that we’ve been talking about today in a way that can unlock the power of the data and actually give people actionable items that they can work on to improve results. What are your clients asking you in this realm? Is the government at that level, are they heading there, or what’s your assessment of that?
Jim Rebesco:
Yeah, absolutely. I mean, I think speaking from my viewpoint, it’s absolutely thrilling to see the kind of proliferation of analytics. And the overall theme we see is that, increasingly, you see more and more people not just kind of trying to consume big data and make sense of it, but people who are actually kind of layering in analytics to kind of start to ask data the questions that are pertinent at the individual user at that individual analyst, that individual person that needs to do his or her job. They’re getting empowered now through this increasing wave of self-service analytics to be able to slice and dice that data, to ask it a question that’s pertinent to him or her in that day and in that moment. And so, that kind of idea of really pushing that barrier to entry down and lowering that skill cap, so you’ve got more tools at hand than just an Excel spreadsheet—I think is one of the really powerful trends that we’re seeing.
If I can be a little bit loose, maybe 10 years ago, we would’ve been talking about big data and we all would’ve been talking about how the insights are just going to fall out of our data once we get enough of it together. I think it’s safe to say that we know today that that’s no longer true, that you do need that kind of analytics piece between the data and decision to really get something useful. And where’s that being done? I mean, obviously there’s been a bunch of people here speaking today about a diverse set of use cases. But again, I like to go back to the idea of providing structure to an increasingly unstructured world, whether that’s satellite imagery or SAR or natural language or stuff on the internet or tabular data. People are computing that they’re getting primitives that are relevant, and then they’re being able to ask those primitive questions, right? And ChatGPT is one very almost like lay person available kind of expression of that, but it’s simply one element, and I think a much broader sweep and theme of people being able to self-serve analytics. To answer their question without a doubt, I think one of the most exciting things in the industry for me.
Moderator:
I see analytics being used in sports shows all the time. They’re talking about averages and the things that really, you could not possibly gather that kind of information at that speed like you can today. And so, I mean, I marvel in politics the same kind of thing. They can use analytics to bifurcate down to the smallest geographic area. So, those are everyday uses that are being used in the public sphere, in the government sphere, the use of analytics is going to maximize any program that any agency can put in place. Do you see it that way?
Jim Rebesco:
Oh, absolutely. And I think that’s a wonderful comparison to draw with sports. And I’ll make a comment there, and then I’ll talk about the government sector. So, if we’re talking about, let’s take baseball 40 years ago, we might’ve described a pitcher in terms of their wins and losses, how many games the pitcher won when they were starting, and how many games they lost. And 40 years of baseball analytics have convinced us of a lot of things. And one of those is that, hey, that’s not really a causal relationship. That is not a predictive measure. And that doesn’t tell us that that pitcher really is any good or any bad. It tells us if they were on a good team or not. And how’d that come about? That came about from a bunch of baseball nerds getting together and really being able to self-serve and crunch some numbers and ask some interesting questions.
The data, and that’s iterated wildly over the last 40 years within the government realm. We’ve talked about NLP a bunch, and that’s, I think absolutely should be top of mind for everybody, but I’ll try to pick a different example to just kind of add some variety. The proliferation of both commercial and government imagery providers over the last 20 years has been remarkable, right? There are more sensors in space now than there were 20 years ago by several orders of magnitude. And what that’s done is that’s generated several orders of magnitude more data. And what we’re seeing in that space, I think, is a really, really rapid evolution of computer vision algorithms and then business logic and business analytics below that to not just structure the world, say, here’s a tree, here’s a house, here’s a car, here’s a road. But then to say, and how many cars are in the parking lot, and when do they come in the morning and when do they leave? And what’s normal and what’s different? And that’s really kind of helping drive scientists, not just kind of get boxes around cars if that was a standard 10 years ago, but get the answers to their questions in an email, in a digest, in a text alert. And I think that’s really kind of where you see the power of the analytics as they start to disappear. And the answers are really what surfaces to the top.
Moderator:
It’s true. And thank you very much, Jim—James—we appreciate that.
Moderator, Question 3:
James, founder of Striveworks. Tell us what you think.
Jim Rebesco:
Yeah, I think there’s been a lot of fun things that have been talked about here in the interest of brevity. I’ll just kind of double-click on this idea that we’ve got a very unique time right now in that we have, there’s a lot of use cases, demand, and excitement around people being able to develop and self-serve AI models. We’ve also got a lot of really powerful technologies and best practices being brought in to make sure that that’s done in a way that is governable and transparent. And I think, there’s just my kind of overall summary is, there’s a really, really unique and exciting opportunity right now to bring those together and realize that kind of business ROI for organizations.
Moderator:
That’s the truth.
Video Summary
This podcast explores the state of AI adoption within the federal government, featuring insights from experts across government agencies, academia, and the private sector, including Striveworks’ CEO and cofounder, Jim Rebesco, as a key industry panelist. Here is a selection of three questions from the conversation that feature Jim’s expertise.
The discussion highlights foundational efforts in data management and emphasizes governance, literacy, and stewardship as critical components for successful AI implementation. Panelists address the importance of transparent, secure, and privacy-conscious AI systems while underscoring workforce development as a pivotal step in equipping both current employees and the next generation with essential AI skills. Collaboration among government, industry, and academia emerges as a key theme, fostering the development of best practices and responsible AI standards.
This podcast comes with an accompanying guide: Federal Agencies Prepare for Advanced AI With Proper Training and Compliance.
Related Resources
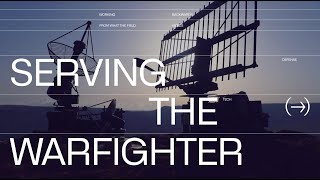
AI-Powered Insight for Mission Critical Operations
Transforming national security with AI, Palantir and Striveworks collaborate to empower decision-makers and deliver mission-critical outcomes.
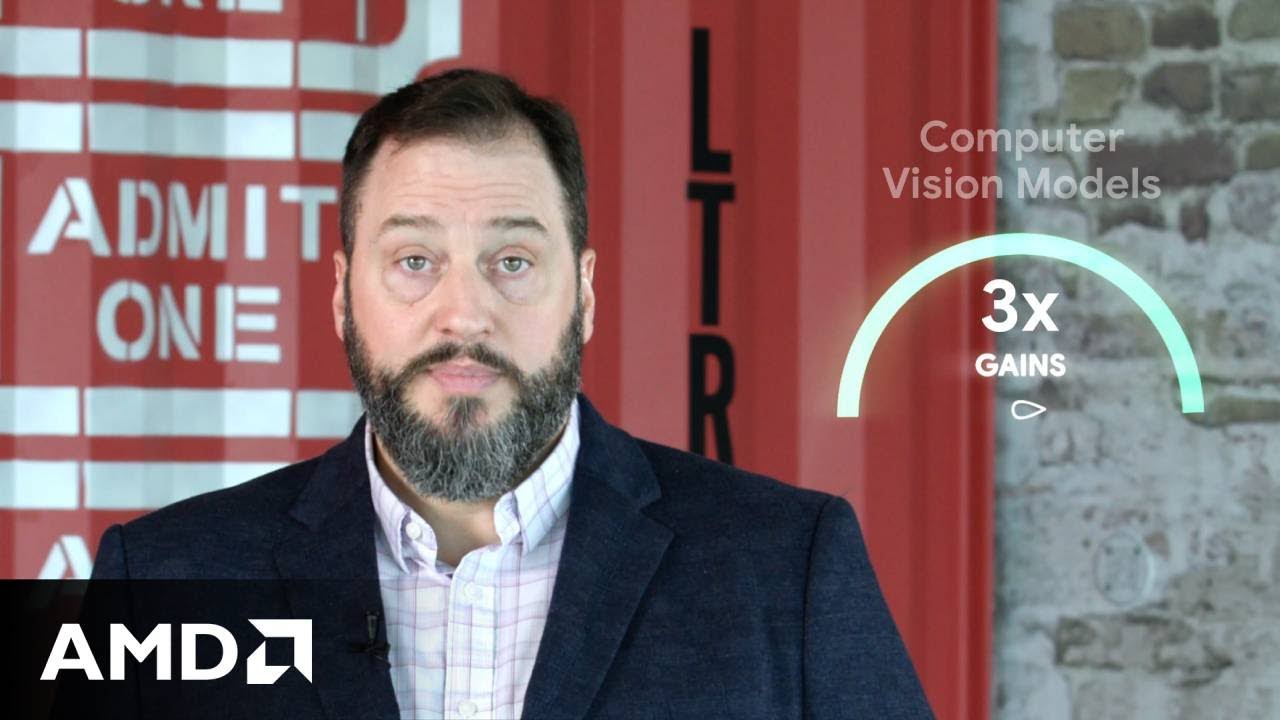
Make AI Accessible for Everyone
Unlock scalable AI effortlessly with Chariot and Striveworks' partners, automating MLOps, reducing costs, and enabling innovation on any infrastructure.