Identify Model Drift and Fast-Track Remediation
Real-world ROI from ML models comes from real-world performance and uptime. Striveworks is the industry-leading solution for keeping models performant in production. Our integrated toolkit streamlines monitoring, evaluation, and retraining to maximize the value of your models throughout their life cycle.
Support Your Post-Production ML Life Cycle
Integrated tools streamline the model remediation process to maximize your AI application's uptime and ROI.
Monitor Production Models
Evaluate and Compare Production Models
Retrain Underperforming Models
Remediate and Redeploy Degraded Models Faster

In a survey, 34% of data professionals reported data integration challenges around retraining and deployment as their #1 pain point.* With Striveworks, data teams can quickly find and use the right production data for model retraining and fine-tuning.
- Dedicated inference store for query and analysis of production inferences by metadata, model performance, and other parameters.
- Automated versioning of datasets to support rapid experimentation.
- Shared user feedback on datasets, such as hard positives and negatives, behaviors, and data labels.
- Integrated model test, evaluation, and comparison to ensure the best model is getting to production.
- Ability to promote models to production and redeploy in one click.
*Source: Thrive in the Digital Era with AI Lifecycle Synergies

Real-Time Automated Monitoring
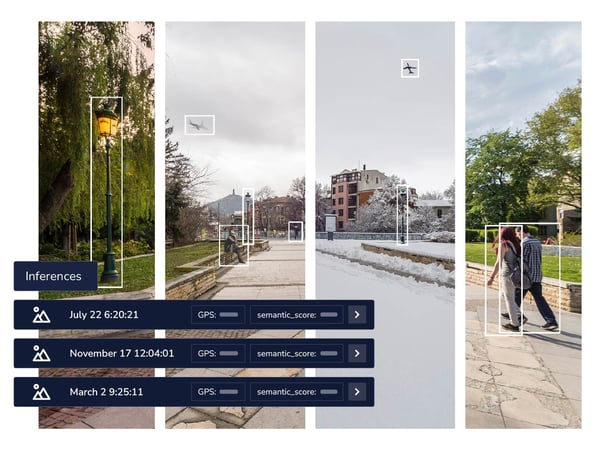
Monitor data drift, model performance, and resource consumption automatically. With Striveworks, you can go beyond basic baselines and trends and compare every incoming data point to model training data, providing a granular history for easy analysis.
- Identify drift in unstructured data—automatically.
- Receive real-time alerts in the platform or through integrations.
- Explore your inference history to find patterns in anomalous data.
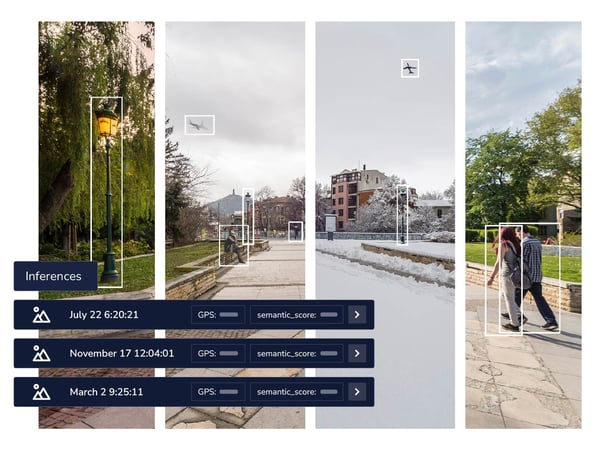
Retrain and Redeploy Models Without Starting From Scratch

Get production data ready for retraining in a fast, traceable, and repeatable manner. Striveworks makes it easy to integrate and curate production data, integrate human feedback on inferences, and isolate the specific failure cohorts of models.
- Filter and curate production data.
- Label and clean production data on the platform for retraining.
- Bootstrap data curation and labeling with human feedback.
- Create new datasets, tracking every split and variation.

Reevaluate Model Performance on Production Data
How much better is your retrained model? Know for certain with Striveworks. Our centralized evaluation store enables fine-grained evaluation of available models to ensure you promote the most suitable options for your real-world applications—systematically and consistently.
- Evaluate model performance against production data.
- Confirm improvements in performance post-retraining.
- Determine the most effective model available for your data pipeline.
Continuously Observe Comprehensive Model Metrics
The Striveworks monitoring dashboard provides a clear-eyed view into performance metrics and key details for your entire model catalog. Track critical metrics that show model efficacy—and give you the knowledge to take action whenever models need attention.
- F1 scores
- Deployment status
- Latency
- Uptime
- Total requests and requests per second
- Data drift metrics
Striveworks MLOps for the Entire Model Life Cycle
The ability to create, find, and use the optimal datasets is critical across every stage of machine learning—including post-production. So, too, is the need to evaluate and observe model behavior.
Striveworks connects all critical data science workflows in an end-to-end system designed for testing and evaluating ML on real and changing data.
Build, Deploy, and Maintain Your Models With Striveworks.
Remediation in Action
In 2022, a Fortune 500 customer engaged Striveworks to build a complex model using data fusion to predict lightning-induced wildfires. When weather conditions changed, incoming data naturally drifted. The Striveworks platform alerted users to model degradation and enabled immediate remediation, allowing the data science team to push an improved version live in hours—delivering 1.1 million predictions in six weeks at 87% accuracy.
Related Resources
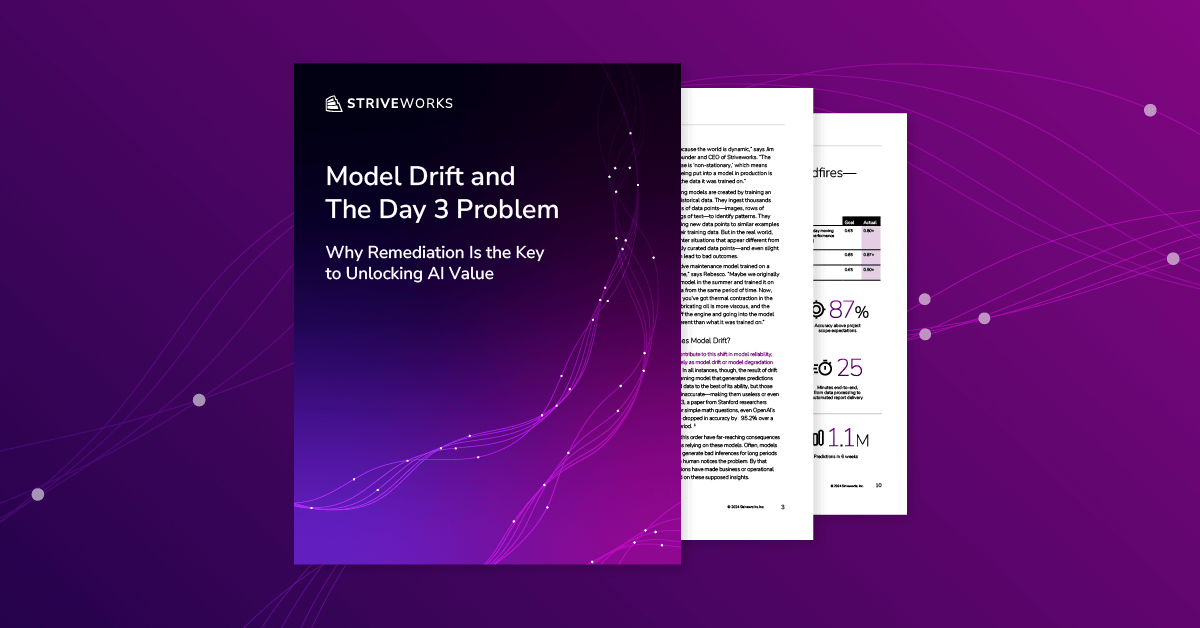
Model Drift and The Day 3 Problem
Model drift is the number one reason AI programs fail to produce value. Fortunately, it doesn’t have to be this way. Remediation is proven to overcome model drift and keep AI projects performant longer. It’s the missing key to unlocking AI’s potential.